It’s not just GPAs
Posted: February 10, 2016 Filed under: Education | Tags: advocacy, aesthetics, ATAR, authenticity, balance, community, education, educational problem, educational research, ethics, fairness, GPA, higher education, resources, student perspective Leave a commentIf you’re watching the Australian media on higher education, you’ll have seen a lot of discussion regarding the validity of the Australian Tertiary Admission Rank (ATAR) as a measure of a student’s future performance and as an accurate summary of the previous years of education.
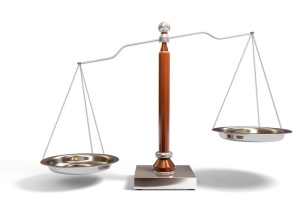
When you are being weighed in the balance, you probably want to know a lot about the scale and the measures.
This article, talking about students being admitted below the cut-offs, contains a lot of discussion on the issue. Not all of the discussion is equally valuable, in my opinion, as the when the question is the validity of the measure, being concerned about ‘standards slipping’ when a lower number is used isn’t that relevant. The interesting parts of the discussion are which mechanisms we should be using and making them transparent so that all students are on a level playing field.
The fact is that students are being admitted to, and passing, courses that have barriers in place which should clearly indicate their chances of success. Yet students are being admitted based on other pathways, using additional measures such as portfolios, and this makes a bit of a mockery on the apparent simplicity of the ATAR system.
My own analysis of student ATAR versus GPA is revelatory: the mapping is a very noisy correlation and, apart from the very highest ATARs, we see people who succeed or fail in a way that does not match their representative ATAR. Yes, there are rough ‘buckets’ but at a granularity of fewer than five buckets, rather than the thousand or so we’re pretending to have.
“Reducing six years of education to a single ranking is simplistic, let’s have a constructive debate about what could replace the ATAR alone as a fairer, more comprehensive and contextual measure of academic potential”.
Iain Martin, from this linked opinion piece.
I couldn’t agree more!
Streamlining for meaning.
Posted: February 9, 2016 Filed under: Education | Tags: advocacy, aesthetics, assessment, authenticity, beauty, competency-based assessment, design, education, educational problem, educational research, ethics, GPA, higher education, in the student's head, reflection, resources, student perspective, teaching, teaching approaches, thinking Leave a commentIn yesterday’s musings on Grade Point Average, GPA, I said:
But [GPA calculation adjustment] have to be a method of avoidance, this can be a useful focusing device. If a student did really well in, say, Software Engineering but struggled with an earlier, unrelated, stream, why can’t we construct a GPA for Software Engineering that clearly states the area of relevance and degree of information? Isn’t that actually what employers and people interested in SE want to know?
This hits at the heart of my concerns over any kind of summary calculation that obscures the process. Who does this benefit? What use it is to anyone? What does it mean? Let’s look at one of the most obvious consumers of student GPAs: the employers and industry.
Feedback from the Australian industry tells us that employers are generally happy with the technical skills that we’re providing but it’s the softer skills (interpersonal skills, leadership, management abilities) that they would like to see more of and know more about. A general GPA doesn’t tell you this but a Software Engineering focused GPA (as I mentioned above) would show you how a student performed in courses where we would expect to see these skills introduced and exercised.
Putting everything into one transcript gives people the power to assemble this themselves, yes, but this requires the assembler to know what everything means. Most employers have neither the time nor inclination to do this for all 39 or so institutions in Australia. But if a University were to say “this is a summary of performance in these graduate attributes”, where the GAs are regularly focused on the softer skills, then we start to make something more meaningful out of an arbitrary number.
But let’s go further. If we can see individual assessments, rather than coarse subject grades, we can start to construct a model of an individual across the different challenges that they have faced and overcome. Portfolios are, of course, a great way to do this but they’re more work to read than single measures and, too often, such a portfolio is weighed against simpler, apparently meaningful measures such as high GPAs and found wanting. Portfolios also struggle if placed into a context of previous failure, even if recent activity clearly demonstrates that a student has moved on from that troubled or difficult time.
I have a deep ethical and philosophical objection to curve grading, as you probably know. The reason is simple: the actions of one student should not negatively affect the outcomes of another. This same objection is my biggest problem with GPA, although in this case the action and outcomes belong to the same student at different points in her or his life. Rather than using performance in one course to determine access to the learning upon which it depends, we make these grades a permanent effect and every grade that comes afterwards is implicitly mediated through this action.

Sometimes you should be cautious regarding adding curves to address your problems.
Should Past Academic Nick have an inescapable impact on Now and Future Academic Nick’s life? When we look at all of the external influences on success, which make it clear how much totally non-academic things matter, it gets harder and harder to say “Yes, Past Academic Nick is inescapable.” Unfairness is rarely aesthetically pleasing.
An excellent comment on the previous post raised the issue of comparing GPAs in an environment where the higher GPA included some fails but the slightly lower GPA student had always passed. Which was the ‘best’ student from an award perspective? Student A fails three courses at the start of his degree, student B fails three courses at the end. Both pass with the same GPA, time to completion, and number of passes and fails. Is there even a sense of ‘better student’ here? B’s struggles are more immediate and, implicitly, concerns would be raised that these problems could still be active. A has, apparently, moved on in some way. But we’d never know this from simplistic calculations.
If we’re struggling to define ‘best’ and we’re not actually providing something that many people feel is useful, while burdening students with an inescapable past, then the least we can do is to sit down with the people who are affected by this and ask them what they really want.
And then, when they tell us, we do something about changing our systems.
Dances with GPAs
Posted: February 8, 2016 Filed under: Education | Tags: advocacy, aesthetics, assessment, authenticity, beauty, community, competency-based assessment, education, educational problem, educational research, ethics, GPA, higher education, in the student's head, resources, student perspective, teaching, thinking, tools 4 Comments
The trick to dancing with dragons is to never lose your grip on the tail.
If we are going to try and summarise a complicated, long-term process with a single number, and I don’t see such shortcuts going away anytime soon, then it helps to know:
- Exactly what the number represents.
- How it can be used.
- What the processes are that go into its construction.
We have conventions as to what things mean but, when we want to be precise, we have to be careful about our definition and our usage of the final value. As a simple example, one thing that often surprises people who are new to numerical analysis is that there is more than one way of calculating the average value of a group of numbers.
While average in colloquial language would usually mean that we take the sum of all of the numbers and divide them by their count, this is more formally referred to as the arithmetic mean. What we usually want from the average is some indication of what the typical value for this group would be. If you weigh ten bags of wheat and the average weight is 10 kilograms, then that’s what many people would expect the weight to be for future bags, unless there was clear early evidence of high variation (some 500g, some 20 kilograms, for example.)
But the mean is only one way to measure central tendency in a group of numbers. We can also measure the median, the number that separates the highest half of the data from the lowest, or the mode, the value that is the most frequently occurring value in the group.
(This doesn’t even get into the situation where we decide to aggregate the values in a different way.)
If you’ve got ten bags of wheat and nine have 10 kilograms in there, but one has only 5 kilograms, which of these ways of calculating the average is the one you want? The mode is 10kg but the mean is 9.5kg. If you tried to distribute the bags based on the expectation that everyone gets 9.5, you’re going to make nine people very happy and one person unhappy.
Most Grade Point Average calculations are based on a simple arithmetic mean of all available grades, with points allocated from 0 to an upper bound based on the grade performance. As a student adds more courses, these contributions are added to the calculation.
In yesterday’s post, I mused on letting students control which grades go into a GPA calculation and, to explore that, I now have to explain what I mean and why that would change things.
As it stands, because a GPA is an average across all courses, any lower grades will permanently drop the GPA contribution of any higher grades. If a student gets a 7 (A+ or High Distinction) for 71 of her courses and then a single 4 (a Passing grade) for one, her GPA will be 6.875. It can never return to 7. The clear performance band of this student is at the highest level, given that just under 99% of her marks are at the highest level, yet the inclusion of all grades means that a single underperformance, for whatever reason, in three years has cost her standing for those people who care about this figure.
My partner and I discussed some possible approaches to GPA that would be better and, by better, we mean approaches that encourage students to improve, that clearly show what the GPA figure means, and that are much fairer to the student. There are too many external factors contributing to resilience and high performance for me to be 100% comfortable with the questionable representation provided by the GPA.
Before we even think about student control over what is presented, we can easily think of several ways to make a GPA reflect what you have achieved, rather than what you have survived.
- We could only count a percentage of the courses for each student. Even having 90% counted means that students who stumble a little once or twice do not have this permanently etched into a dragging grade.
- We could allow a future attempt at a course with an improved to replace the previous grade. Before we get too caught up in the possibility of ‘gaming’, remember that students would have to pay for this (even if delayed) in most systems and it will add years to their degree. If a student can reach achievement level X in a course then it’s up to us to make sure that does correspond to the achievement level!
- We could only count passes. Given that a student has to assemble sufficient passing grades to be awarded a degree, why then would we include the courses that do not count in a calculation of GPA?
- We could use the mode and report the most common mark the student receives.
- We could do away with it totally. (Not going to happen any time soon.)
- We could pair the GPA with a statistical accompaniment that tells the viewer how indicative it is.
Options 1 and 2 are fairly straight-forward. Option 3 is interesting because it compresses the measurement band to a range of (in my system) 4-7 and this then implicitly recognises that GPA measures for students who graduate are more likely to be in this tighter range: we don’t actually have the degree of separation that we’d assume from a range of 0-7. Option 4 is an interesting way to think about the problem: which grade is the student most likely to achieve, across everything? Option 5 is there for completeness but that’s another post.
Option 6 introduces the idea that we stop GPA being a number and we carefully and accurately contextualise it. A student who receives all high distinctions in first semester still has a number of known hurdles to get over. The GPA of 7 that would be present now is not as clear an indicator of facility with the academic system as a GPA of 7 at the end of a degree, whichever other GPA adjustment systems are in play.
More evidence makes it clearer what is happening. If we can accompany a GPA (or similar measure) with evidence, then we are starting to make the process apparent and we make the number mean something. However, this also allows us to let students control what goes into their calculation, from the grades that they have, as a clear measure of the relevance of that measure can be associated.
But this doesn’t have to be a method of avoidance, this can be a useful focusing device. If a student did really well in, say, Software Engineering but struggled with an earlier, unrelated, stream, why can’t we construct a GPA for Software Engineering that clearly states the area of relevance and degree of information? Isn’t that actually what employers and people interested in SE want to know?
Handing over an academic transcript seems to allow anyone to do this but human cognitive biases are powerful, subtle and pervasive. It is harder for most humans to recognise positive progress in the areas that they are interested in, if there is evidence of less stellar performance elsewhere. I cite my usual non-academic example: Everyone thought Anthony La Paglia’s American accent was too fake until he stopped telling people he was Australian.
If we have to use numbers like this, then let us think carefully about what they mean and, if they don’t mean that much, then let’s either get rid of them or make them meaningful. These should, at a fundamental level, be useful to the students first, us second.
The Part and the Whole
Posted: October 13, 2014 Filed under: Education | Tags: advocacy, analytics, authenticity, computer science, computer science education, GPA, learning, learning analytics, student, synecdoche, teaching, thinking Leave a commentI like words a lot but I also love words that introduce me to whole new ways of thinking. I remember first learning the word synecdoche (most usually pronounced si-NEK-de-kee), where you used the word for part of something to refer to that something as a whole (or the other way around). Calling a car ‘wheels’ or champagne ‘bubbles’ are good examples of this. It’s generally interesting which parts people pick for synecdoche, because it emphasises what is important about something. Cars have many parts but we refer to it in parts as wheelsI and motor. I could bore you to tears with the components of champagne but we talk about the bubbles. In these cases, placing emphasis upon one part does not diminish the physical necessity of the remaining components in the object but it does tell us what the defining aspect of each of them is often considered to be.
There are many ways to extract a defining characteristic and, rather than selecting an individual aspect for relatively simple structures (and it is terrifying that a car is simple in this discussion), we use descriptive statistics to allow us to summarise large volumes of data to produce measures such as mean, variance and other useful things. In this case, the characteristic we obtain is not actually part of the data that we’re looking at. This is no longer synecdoche, this is statistics, and while we can use these measures to arrive at an understanding (and potentially move to the amazing world of inferential statistics), we run the risk of talking about groups and their measurements as if the measurements had as much importance as the members of the group.
I have been looking a lot at learning analytics recently and George Siemens makes a very useful distinction between learning analytics, academic analytics and data mining. When we analyse the various data and measures that come out of learning, we want to use this to inform human decision making to improve the learning environment, the quality of teaching and the student experience. When we look at the performance of the academy, we worry about things like overall pass rates, recruitment from external bodies and where our students go on to in their careers. Again, however, this is to assist humans in making better decisions. Finally, and not pejoratively but distinctly, data mining delves deep into everything that we have collected, looking for useful correlations that may or may not translate into human decision making. By separating our analysis of the teaching environment from our analysis of the academic support environment, we can focus on the key aspects in the specific area rather than doing strange things that try to drag change across two disparate areas.
When we start analysis, we start to see a lot of numbers: acceptable failure rates, predicted pass rates, retention figures, ATARs, GPAs. The reason that I talk about data analytics as a guide to human decision making is that the human factor reminds us to focus on the students who are part of the figures. It’s no secret that I’m opposed to curve grading because it uses a clear statement of numbers (70% of students will pass) to hide the fact that a group of real students could fail because they didm’ perform at the same level as their peers in the same class. I know more than enough about the ways that a student’s performance can be negatively affected by upbringing and prior education to know that this is not just weak sauce, but a poisonous and vicious broth to be serving to students under the guide of education.
I can completely understand that some employers want to employ people who are able to assimilate information quickly and put it into practice. However, let’s be honest, an ability to excel at University is not necessarily an indication of that. They might coincide, certainly, but it’s no guarantee. When I applied for Officer Training in the Army, they presented me with a speed and accuracy test, as part of the battery of psychological tests, to see if I could do decision making accurately at speed while under no more stress than sitting in a little room being tested. Later on, I was tested during field training, over and over again, to see what would happen. The reason? The Army knows that the skills they need in certain areas need specific testing.
Do you want detailed knowledge? Well, the numbers conspire again to undermine you because a focus on numerical grade measures to arrive at a single characteristic value for a student’s performance (GPA) makes students focus on getting high marks rather than learning. The GPA is not the same as the wheels of the car – it has no relationship to the applicable ability of the student to arbitrary tasks nor, if I may wax poetic, does it give you a sense of the soul of the student.
We have some very exciting tools at our disposal and, with careful thought and the right attitude, there is no doubt that analytics will become a valuable way to develop learning environments, improve our academies and find new ways to do things well. But we have to remember that these aggregate measures are not people, that “10% of students” represented real, living human beings who need to be counted, and that we have a long way to go before have an analytical approach that has a fraction of the strength of synecdoche.